PROJECT OVERVIEW
Fitting physical models with spatial data
In the study of natural hazards, we often use field measurements to calibrate models of physical processes and estimate source parameters. Through connected works, we critically examine the assumptions behind this practice and propose methods to better reflect reality.
Loss functions and varying data uncertainties in model calibration: the case of TEPHRA2 inversion
Team: Maricar Rabonza, Michele Nguyen, Sebastien Biasse, Susanna Jenkins, David Lallemant
Probabilistic calibration of a flood simulator using spatially distributed binary observations
Team: Mariano Balbi, David Lallemant
Loss functions and varying data uncertainties in model calibration: the case of TEPHRA2 inversion
To estimate the source parameters that describe the dynamics of a volcanic eruption, field measurements of the tephra deposit are often used to calibrate forward models through a process known as inversion. In this work, we look at specific features of the observed data that are often overlooked in both the inversion of the eruption source parameters as well as the forward estimation in spatial distribution of tephra. These, as well as the proposed strategies to address them, are as follows
Uncertainty in the tephra load/depth observations
In inversion, uncertainty in tephra measurements can be accounted for by data-weighting.
Distribution of the residuals
The choice of loss function has significant impact in the inversion results, and this choice can be informed through analysis of the model residuals.
Residual spatial correlation
Both the uncertainty in tephra measurements and their spatial correlation can be used in the prediction of the full spatial distribution of tephra depth through various spatial statistical methods.
This project aims to provide guidance to modelers conducting inversion of eruption source parameters and/or using the source parameters to conduct a forward estimate of the spatial distribution of tephra deposit. While the study is focused on a case study and model for tephra inversion, the takeaways are broadly applicable to other environmental modelling settings involving calibration and inversion.
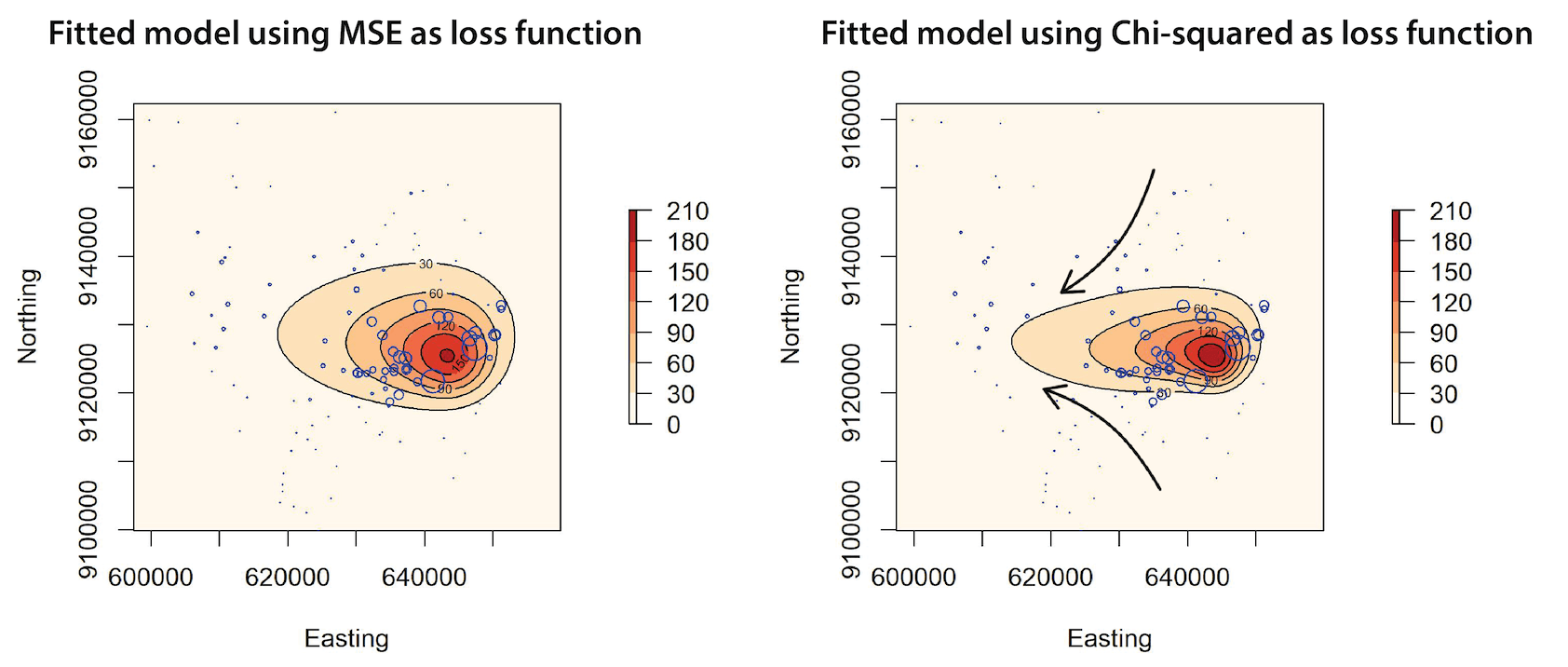
Figure 1: Effect of a relative loss function on a fitted forward model.
The prediction using Chi-squared as loss function elongates to better fit the smaller valued observations further away from the vent. A relative loss function allows small values to speak out more. This could be important in the context of tephra deposits where small values further away from the vent could tell us about grain size distribution. Weights were not applied to the fitted models shown.
Probabilistic calibration of a flood simulator using spatially distributed binary observations
Flood simulators are complex and spatially explicit computational models that aim to represent the dynamics of the water as it propagates through rivers and floodplains. To obtain meaningful inferences from its outputs, proper calibration of model parameters should be done based on real-world observations and expert knowledge.
This work provides a robust framework to obtain calibrated predictions from the flood simulator conditioned on spatially distributed binary observations of flood extent, as obtained from SAR satellite imagery, using a spatially dependent probit model. Focus is made on the characterization of the different uncertainty sources that influence inference, including
Parameter uncertainty
Observation error
Structural inadequancy of the simulator
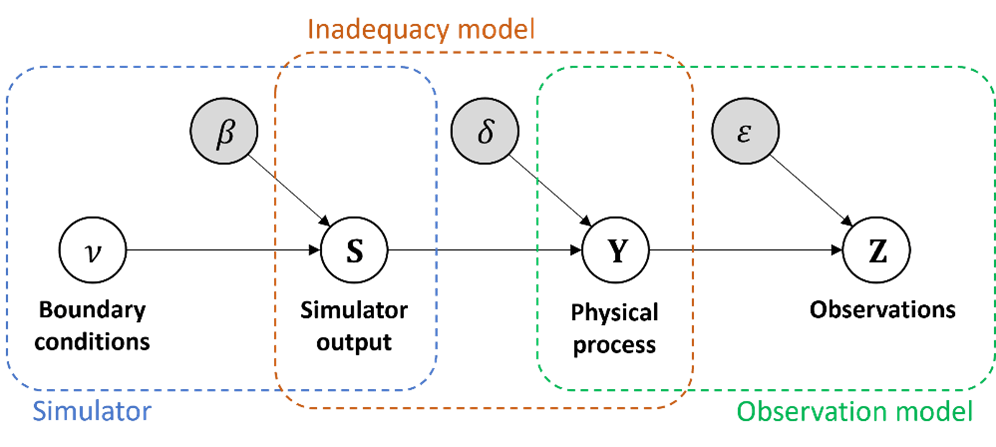
Figure 2: Correlation structure of uncertainty framework for computational simulators.